What is Deep Learning? A Comprehensive Guide to Understanding AI’s Core Technology
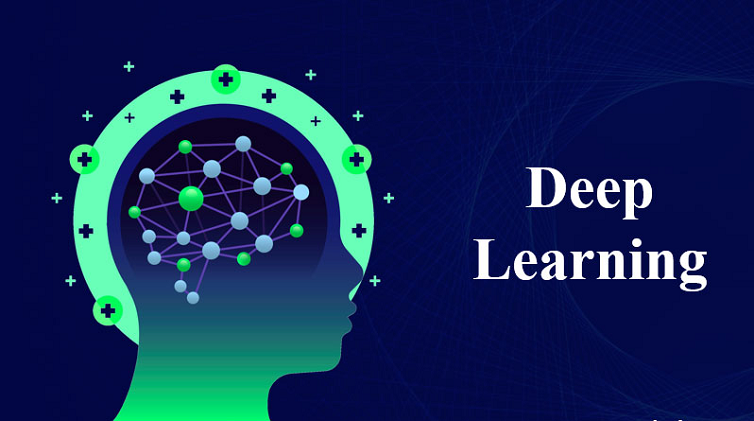
Deep learning is at the heart of modern artificial intelligence (AI), powering showbizztoday.com transformative technologies that redefine how humans interact with machines. From facial recognition on smartphones to self-driving cars, solid Learning has become the backbone of innovative solutions in multiple industries. But what exactly is deep learning, and why is it so powerful?
In this article, we explore deep learning in detail. You’ll learn its definition, underlying principles, how it works, and why it’s so impactful. We’ll also cover its applications, advantages, challenges, and future potential. If you’re seeking a clear understanding of deep learning, this guide is for you.
What is Deep Learning?
Deep learning is a subset of machine learning, which itself is a branch of artificial intelligence. It involves training computers to mimic the way humans learn through the use of artificial neural networks. These neural networks are inspired by the structure and functioning of the human brain, enabling machines to analyze and process large amounts of data efficiently.
Unlike traditional machine learning, which often requires manual feature extraction, solid Learning models can automatically identify patterns and relationships in data. This makes it particularly effective for complex tasks such as image recognition, natural language processing, and speech recognition.
How Does Deep Learning Work?
Deep learning operates on a hierarchical structure of artificial neural networks. These networks consist of multiple layers of interconnected nodes or “neurons.” Each layer processes data and passes the output to the next layer, gradually learning more abstract features at each stage.
Input Layer
The input layer receives raw data, such as images, text, or audio. This data is preprocessed and transformed into numerical form for further analysis.
Hidden Layers
The hidden layers perform the heavy lifting. Each hidden layer extracts specific features from the data, with deeper layers uncovering more complex patterns. These layers use mathematical functions to identify relationships and assign weights to connections.
Output Layer
The final layer produces the output, which can be a classification, prediction, or decision based on the task at hand. For example, it might identify whether an image contains a cat or a dog.
Deep learning models rely on vast amounts of data and computational power to achieve accuracy. Training involves iterative adjustments to the weights and biases of the neurons to minimize errors in predictions—a process known as backpropagation.
Key Characteristics of Deep Learning
Deep learning has several defining characteristics that make it stand out:
- Feature Learning
Deep learning models automatically extract features from data, eliminating the need for manual feature engineering. - Scalability
These models scale effectively with large datasets, improving performance as more data is provided. - Nonlinear Processing
Deep learning networks can model nonlinear relationships, making them suitable for complex data patterns. - End-to-End Learning
Deep learning enables end-to-end learning, where raw data is processed directly to generate output without intermediate steps.
Applications of Deep Learning
Deep learning has revolutionized numerous industries, offering solutions to problems that were previously unsolvable. Here are some of its most impactful applications:
Computer Vision
Deep learning excels in tasks like image recognition, object detection, and facial recognition. It’s widely used in applications such as autonomous vehicles, medical imaging, and security systems.
Natural Language Processing (NLP)
NLP is another area where solid Learning has made significant advancements. Chatbots, virtual assistants, sentiment analysis, and language translation tools rely on deep learning algorithms.
Speech Recognition
Deep learning powers voice-activated systems like Siri, Alexa, and Google Assistant. It enables machines to understand and process spoken language with high accuracy.
Healthcare
In the healthcare industry, deep learning assists in diagnosing diseases, analyzing medical images, and predicting patient outcomes. It’s also used in drug discovery and personalized medicine.
Autonomous Vehicles
Self-driving cars depend on deep learning to interpret sensor data, identify objects, and make real-time decisions for safe navigation.
Finance
In finance, deep learning models are used for fraud detection, algorithmic trading, and risk assessment.
Gaming and Entertainment
Deep learning is used in game development for creating realistic graphics, simulating physics, and generating intelligent non-player characters (NPCs).
Advantages of Deep Learning
Deep learning offers numerous benefits, making it a preferred approach for solving complex problems:
- High Accuracy
Deep learning models achieve exceptional accuracy in tasks like image and speech recognition. - Automation
The ability to automate feature extraction reduces the need for manual intervention, saving time and resources. - Adaptability
These models can adapt to new data and learn continuously, improving their performance over time. - Versatility
Deep learning is versatile and can be applied to a wide range of problems across different domains.
Challenges of Deep Learning
While deep learning is a powerful tool, it comes with its own set of challenges:
- Data Requirements
Deep learning requires vast amounts of labeled data for training, which can be difficult to obtain. - Computational Costs
Training deep learning models demands significant computational power, often requiring specialized hardware like GPUs. - Interpretability
Deep learning models are often considered “black boxes,” making it difficult to understand how they arrive at specific decisions. - Overfitting
When a model learns too much from the training data, it may perform poorly on unseen data. - Ethical Concerns
The use of deep learning in surveillance, privacy invasion, and biased decision-making raises ethical questions.
Deep Learning vs. Machine Learning
Although deep learning is a subset of machine learning, there are key differences between the two:
Feature | Machine Learning | Deep Learning |
---|---|---|
Feature Engineering | Manual feature extraction | Automatic feature extraction |
Data Requirements | Works with small datasets | Requires large datasets |
Complexity | Handles simple and structured data | Excels with complex and unstructured data |
Training Time | Faster training | Slower due to complex networks |
Interpretability | Easier to interpret | Harder to interpret |
Frequently Asked Questions (FAQs)
Q1. What is deep learning in simple terms?
Deep learning is a type of artificial intelligence that trains machines to mimic human learning using artificial neural networks. It’s used for tasks like image recognition, speech processing, and language translation.
Q2. How is deep learning different from machine learning?
Deep learning is a subset of machine learning that uses neural networks to automatically extract features from data, whereas machine learning often requires manual feature engineering.
Q3. What are some real-life applications of deep learning?
Deep learning is used in various industries, including self-driving cars, virtual assistants, medical imaging, fraud detection, and gaming.
Q4. Why does deep learning require so much data?
Deep learning models learn from patterns in data. The more data they are exposed to, the better they can generalize and perform accurately on new tasks.
Q5. What are the challenges of deep learning?
Challenges include high computational costs, large data requirements, difficulty in interpretation, risk of overfitting, and ethical concerns.
Q6. Can deep learning replace human intelligence?
Deep learning is designed to replicate specific human tasks but lacks general intelligence. It complements human capabilities rather than replacing them.
The Future of Deep Learning
The future of deep learning is bright, with advancements expected in areas such as unsupervised learning, explainable AI, and quantum computing. As deep learning becomes more efficient and accessible, its applications will expand further, transforming industries and reshaping the way humans interact with technology.
Companies and researchers are also working on reducing the computational and data demands of deep learning, making it more sustainable and ethical. Emerging fields like neuromorphic computing and edge AI are poised to accelerate this transformation.
In conclusion, deep learning represents a significant leap forward in artificial intelligence. Its ability to solve complex problems with high accuracy has made it indispensable in today’s world. For businesses, professionals, and enthusiasts, understanding solid Learning is essential to staying ahead in the tech-driven landscape.